In the first proof-of-concept, technology for self-driving cars, imec specifically focuses on AI solutions that allow self-driving cars to get a better understanding of their surroundings. Starting with better object recognition. With the current status of the technology, other road users are sometimes still incorrectly identified. For example, when a car is driving on the pavement, it could fall outside the framework of what the software has learned in terms of pattern recognition. Next, also being able to predict traffic situations and intentions of other road users come into play.
Think of anticipating a cyclist making a turn, because he or she is already slightly deviating or the cyclist is looking in a certain direction.
As human drivers we can interpret such subtle signals, but for the moment self-driving cars cannot. The results of this case can also be applied in other industrial environments where self-driving vehicles are used, such as logistics or agriculture. Or in situations where human intentions must be interpreted, such as crowd management or healthcare.
In the second case, the interaction of drivers with their car, imec is working on technology that allows for complex conversations between man and machine.
Compare it to a digital taxi driver. You can not only instruct him to take you from A to B, but you can also ask for all kinds of other information along the way.
For example: "Why is it so busy here?" "Because there is a manifestation." "From whom?" "The unions are on strike today." "Why?" "Better working conditions in the catering industry" "Will there be a restaurant open tonight?" " Sure, what do you want to eat?" etc... The technology necessary to enable such advanced conversations goes beyond the pattern-based schemes behind current chatbots that are bound to a specific context. Once developed, it will also be useful for applications in retail, healthcare, etc.
The third scenario, assisting operators, requires an even more advanced development of the conversation between man and machine. This scenario focuses on situations in which operators perform actions with a low degree of repetition and which are therefore difficult to predict. In these situations, imec wants to develop technology that can help the operator to perform the work effectively and safely. Think of suggestions about a correct posture when lifting heavy loads, the correct use of hazardous machines, etc.
An AI assistant could give tips and instructions by observing the operator. Where possible also fueled by the knowledge it has gained from the observation of other operators.
"Have you ever tried to do this in a different way? I observed that other operators perform it faster or more safely." This type of technology can be used not only in the context of operators, but wherever human actions can be optimized.
To realize these proof-of-concepts, disciplines such as Natural Language Processing, Computer Vision, Cognitive Architectures, Reinforcement & Fast Learning and Recommendation Systems come together. To this end, imec works closely with partners in the AI impulse program, such as the University of Antwerp, KU Leuven and VUB.
Want to know more?
- Learn more about the challenges in the domain of Human-like AI in the article: Tower of Babel: Human-like AI.
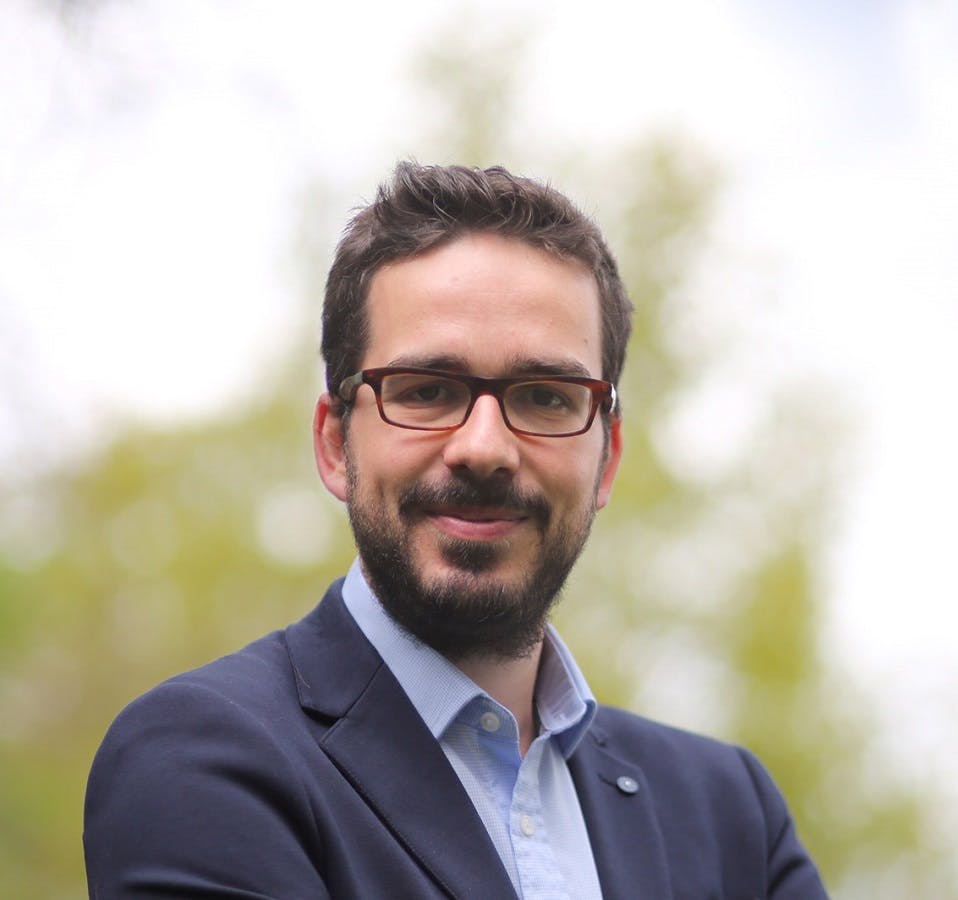
Prof. Steven Latré, is an associate professor at the University of Antwerp and director at the research centre imec, Belgium. He is leading the IDLab Antwerp research group (85+ members), which is performing applied and fundamental research in the area of communication networks and distributed intelligence. His personal research interests are in the domain of machine learning and its application to wireless network optimization.
He received a Master of Science degree in computer science from Ghent University, Belgium and a Ph.D. in Computer Science Engineering from the same university with the title “Autonomic Quality of Experience Management of Multimedia Services”. He is author or co-author of more than 100 papers published in international journals or in the proceedings of international conferences. He is the recipient of the IEEE COMSOC award for best PhD in network and service management 2012, the IEEE NOMS Young Professional award 2014 and is a member of the Young Academy Belgium.
More about these topics:
Published on:
13 May 2019