Next-gen AI, next-gen challenges
Simply adding brute compute power and data has done an excellent job for the Large Language Models. Adding more GPUs, data, and training time, et voilà: model x.0 was born. But as we go beyond generative AI towards reasoning models, workloads become increasingly heterogeneous. Agentic AI, which focuses on decision-making and is highly relevant for medical applications, and physical AI, which focuses on emphasizing embodiment and interaction with the physical world for robotics and autonomous cars, require a myriad of different models. Each model serves a specific purpose and interacts with the others, forming an AI system that can combine large language models, perception models, and action models. Some models require CPUs, some GPUs, and others are currently lacking the right processors. This observation prompted NVIDIA’s Jensen Huang to state that we would eventually needa three-computer solution.
It is clear that a classic one-size-fits-all approach, which only increases compute power, won’t suffice to deal with a chain of various workloads. AI has already become a significant energy consumer, comparable in size to Japan, and the demand for resources is likely to continue growing with the arrival of next-gen AI. The root of the problem is that AI is often running on a suboptimal compute architecture, consisting of suboptimal hardware components for the specific workload the algorithms need. Adding new, challenging workloads to the mix will cause AI-related energy use to rise exponentially. Making it even more challenging is the fact that AI workloads could change overnight, instigated by a new algorithm. Remember the story of the Chinese start-up company, Deepseek, which sent a massive shockwave through the entire industry, and was replicated in less than two weeks by other labs? Indeed, algorithms move quickly. But hardware problems are time-intensive, taking several years to achieve even minor improvements, all the while production is becoming increasingly complex and therefore expensive.
In other words, the tech industry is dealing with a synchronization issue. Developing a specific computing chip for each model, as we do today for generative AI, can't keep up with the unprecedented pace of innovation in models. Furthermore, the laws of economics are not playing in hardware’s favor either: there is a huge inherent risk of stranded assets because by the time the AI hardware is finally ready, the fast-moving AI software community may have taken a different turn. One way AI companies can speed up the development time of AI hardware is to do it themselves: OpenAI starts developing their own custom AI-chips with TSMC. However, this remains a costly and risky endeavor, especially for companies that can put less weight and money on the scale.
Flex is key
It is particularly difficult to predict the next requirement or bottleneck in the rapidly evolving world of AI. One thing we know for sure is that in the long run, flexibility will be key. Silicon hardware should become almost as ‘codable’ as software is. The same set of hardware components should become reconfigurable, meaning you would only need one computer instead of three to run a sequence of algorithms. In fact, software should define silicon, which is a very different approach to current hardware innovation, which is a fairly stiff process.
Picture it: rather than one monolithic ‘state-of-the-art’ and super-expensive processor, you would get different coworking supercells consisting of stacked layers of semiconductors, each optimized for specific functionalities, and integrated in 3D so memory can be placed close to the logic processing unit, thereby limiting the energy losses of data traffic. A network-on-chip will steer and reconfigure these supercells so they can be quickly adapted to the latest algorithm requirements, smartly combining the different versatile building blocks. By splitting up the requirements over different chiplets instead of designing a monolithic chip, you can combine hardware from different suppliers.
This way, new emerging memory technologies that are better suited for AI workloads become possible. For example, imec’s spin-off Vertical Compute integrates vertical data lanes directly on top of the compute units, significantly reducing the distance data must travel from centimeters to nanometers. This approach minimizes data movement, resulting in energy savings of up to 80 percent. It’s one of the many ways in which hardware innovation will enable next-gen AI.
With this reconfigurable approach, many more companies will have the ability to design their own hardware for specific AI workloads. It will boost creativity in the market, open possibilities for differentiation, and make hardware innovation affordable again. By agreeing on a universally accepted standard, like RISC-V, software and hardware companies are getting in sync and guaranteeing both compatibility and performance.
The imec pilot line within the European NanoIC-project is the European answer to AI-driven complexity and further strengthens Europe’s leadership in research by bridging the gap from lab to fab. At the same time, the pilot line will foster a European industry ecosystem of start-ups, AI companies, chip designers, manufacturers, and others around the most advanced technology.
AI’s future hinges on hardware innovations. And given the vast impact of AI on all societal domains, ranging from in silico drug design to sensor fusion for robotics and autonomous driving, it is probably not exaggerated to state that our very future hinges on it.
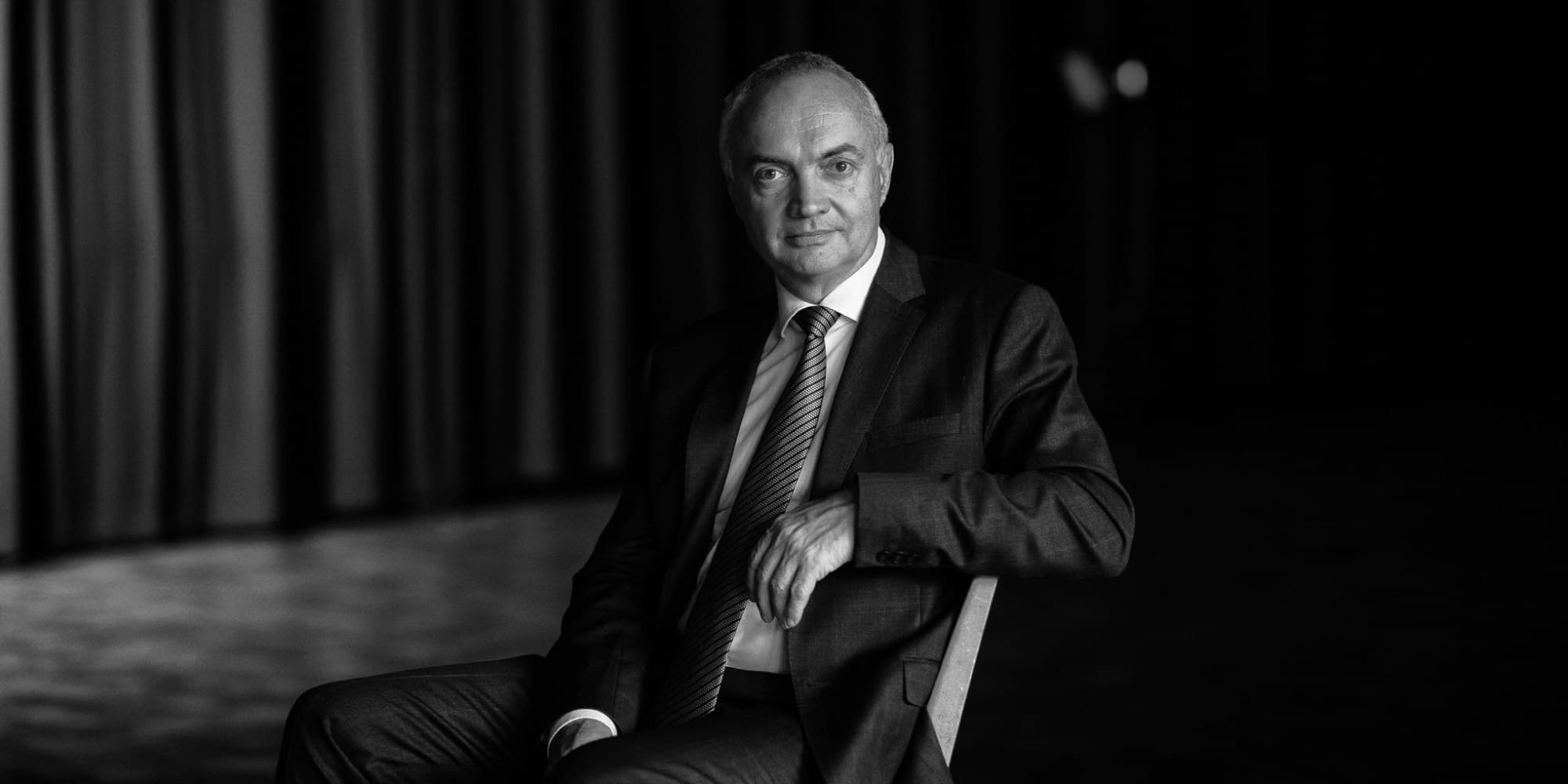
Luc Van den hove has been imec’s president and CEO since July 1, 2009. Prior to that, he served as the research center’s executive vice president and chief operating officer. He joined imec in 1984, starting his research career in the field of silicide and interconnect technologies. Luc Van den hove received his Ph.D. in electrical engineering from the KU Leuven, Belgium. He has authored or co-authored more than 200 publications and conference contributions.
Published on:
22 May 2025