Our computational power has risen exponentially, enabling the widespread use of artificial intelligence (AI), a technology that relies on processing huge amounts of data to recognize patterns. When we use the recommendation algorithm of our favorite streaming service, we usually don't realize the gigantic energy consumption behind it. The billions of operations needed to process the data are typically carried out in datacenters. All these computations together consume a tremendous amount of electric power. Although datacenters heavily invest in renewable energy, a significant part of the power still relies on fossil fuels. The popularity of AI applications clearly has a downside: the ecological cost.
To get a better understanding of the total footprint, we should take two factors into account: training and inference. First, an AI model needs to be trained by a labeled dataset. The ever-growing trend towards the use of bigger datasets for this training phase causes an explosive growth in energy consumption. Researchers from the University of Massachusetts calculated that during the training of a model for natural language processing 284 metric tons of carbon dioxide is emitted. This is equivalent to the emission of five cars during their entire lifespan, including construction. Some AI models developed by tech giants – which are not reported in scientific literature - might be even orders of magnitude bigger.
The training phase is just the beginning of the AI model’s lifecycle. Once the model is trained, it is ready for the real world: finding meaningful patterns in new data. This process, called inference, consumes yet more energy. Unlike training, inference is not a one-off. Inference is taking place continuously. For example, every time a voice assistant is asked a question and generates an answer, extra carbon dioxide is released. After about a million of inference events, the impact will surpass that of the training phase. This process is unsustainable.
Today, both training and inference are typically performed in datacenters. This means beyond the energy involved in the calculations, we should take into account the transmission energy of sending data from the device to the datacenter, and back. Can we avoid part of that traffic by porting the inference process to the device where the data is captured? Besides saving a lot of energy, we could also save time in those cases where immediate decisions are of vital importance, e.g. image classification in selfdriving cars. Decentralizing the data processing would also be a good idea in terms of privacy and security: if your personal information never leaves your phone, it cannot be intercepted. And by bringing the intelligence to the data collecting device, you do not even need an internet connection. So, what is keeping us from implementing this on-device inference? Well, the inference processors today are just too power-hungry for the use in battery-powered edge devices because they were designed first for performance and precision instead of energy-efficiency.
The silver lining: research in developing completely new hardware architectures, aimed at drastically increasing energy efficiency, is picking up very quickly. Pathfinding is done in new Compute-in-Memory architectures, exploiting the most advanced logic and memory components. Recently, imec demonstrated an Analog Inference Accelerator, achieving 2,900 trillion operations per Joule – which is already ten times more energy efficient than today’s digital accelerators. With these types of hardware innovations, it will become possible to directly process data in battery-powered devices – including drones and vehicles –to avoid transmission energy. However, developing energy-efficient hardware is only one side of the solution.
Running inefficient algorithms on an energy-efficient accelerator will wipe out the hardware’s benefits. Therefore, we also need to develop energy-efficient algorithms. This is not only necessary for on-device inference, but also to reduce the number of calculations during inference or training of AI algorithms in datacenters. To solve for this, we can draw inspiration from our own nature: if you are a good tennis player, learning how to play squash is only a small step. Similarly, we can transfer an existing AI model trained in one domain to an adjacent one. After training, we can further minimize the number of calculations by applying compression strategies. The most appealing one is the technique of network pruning: we 'prune out' all the parameters that have little importance for the end result. What remains is an algorithm that has the same functionality, but is smaller, faster and more energy efficient. With the help of this compression strategy, the number of calculations can already be reduced by 30 to 50 percent. Thereafter, more application-based techniques will help us to further improve the efficiency. As such, we can already regain more than 90 percent of power by just optimizing the AI model, apart from the hardware considerations.
We can further improve the efficiency of the algorithms by adapting them to the specificity of the hardware. We can work on both developments independently, but the biggest gain is in the co-optimization. To create truly energy-efficient AI systems, we need an integrated approach that tunes innovations in data usage, hardware, and software.
While the AI research community strongly focusses on these innovations, consumers have no way to figure out how ‘green’ the AI systems they use every day actually are. Awareness would increase if the industry provided estimates of the carbon emission related to the use of a recommendation or image recognition algorithm. Policymakers have introduced energy labels for household appliances, vehicles, and buildings, nudging more investments in energy efficiency. Introducing energy labels for AI-driven applications and systems could have a similar effect.
Note: This article has previously been published on the Forbes Technology Council blog.
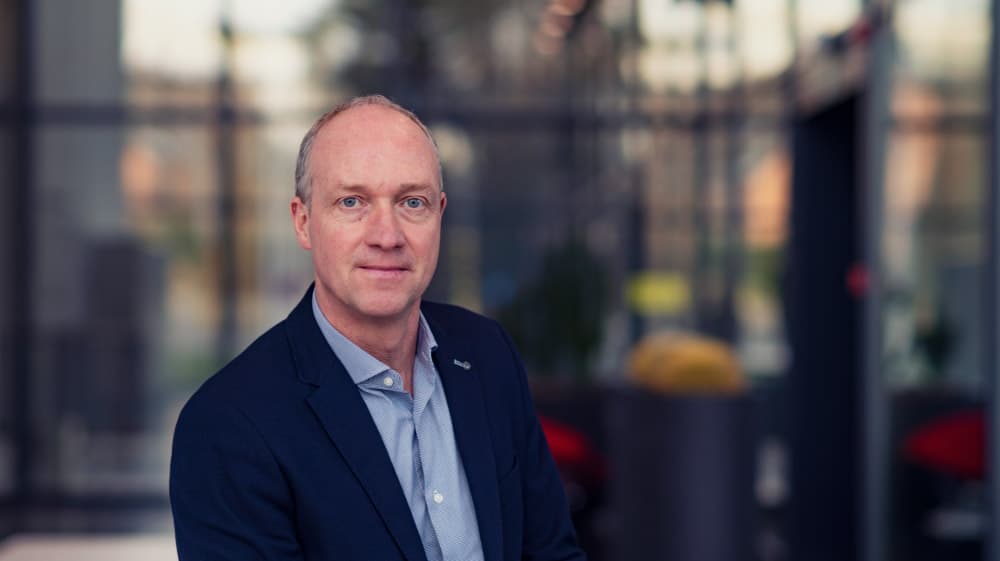
Jo De Boeck joined the company in 1991 after earning his Ph.D. from KU Leuven. He has held various leadership roles, including head of the Microsystems division and CTO. He is also a part-time professor at KU Leuven and was a visiting professor at TU Delft. Jo oversees imec's strategic direction and is a member of the Executive Board.
Published on:
15 February 2021