Complications caused by premature birth are the global leading cause of death in children under five. Yet, according to the World Health Organization, three quarters of these deaths could be avoided by known and relatively inexpensive measures. One of these measures is the administration of corticosteroids to promote fetal lung maturation in case of an expected premature birth. However, since this treatment seems to be most effective between two and seven days before delivery, good timing and a correct assessment are important. Aspects for which even specialized doctors give themselves a lot of room for improvement. That is why an AI algorithm was developed at IDLab, an imec research group at the University of Ghent, which aims to support the medical staff in estimating the risk of premature birth.
What makes the system unique, is that it not only takes the structured data from the medical records (temperature, blood pressure...), but it also analyses the unstructured data (doctors’ notes).
Which is far from straightforward, since not every doctor documents his findings in the same way. For example, some write everything in full, while others use abbreviations. By combining the information from structured and unstructured data, the best results were achieved.
The accuracy of the predictions, compared to non-software- assisted ones, was increased by ten percent or more.
The collaboration between the physician and the algorithm remains vital. Both of them make a prediction and the cases in which they disagree are the most interesting ones. The algorithm is transparent and indicates which data it uses to draw its conclusion. It is then up to the doctor to look at this AI-reasoning and make the final decision. This way, a two-way learning is established. Doctors are provided with more knowledge, which can help them to more quickly and correctly assess potential premature birth and handover the patient in case the delivery has to take place in a specialized center. The other way around, they improve the algorithm by correcting it when their personal experience prevails over the reasoning and conclusion of the software.
The project is a close collaboration between IDlab, an imec research group at Ghent University and the Ghent University Hospital (UZ Gent). The current software was successfully applied to an existing dataset of past premature births. This made it possible to test the accuracy of the predictions because neither the doctors involved nor the algorithm were informed of the outcomes in advance.
In a next step, the predictive power will be tested with new patients and therefore also with an unknown outcome for the researchers.
Also, IDLab is exploring a collaboration with East Limburg Hospital (ZOL) and imec spin-off Bloomlife, which has developed a wearable for home monitoring of contractions. Together they will investigate if the sensor data of this wearable can further improve the accuracy of the AI-algorithm.
Want to know more?
- Homepage of IDLab, an imec research group at Ghent University.
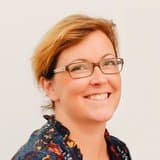
Sofie Van Hoecke graduated from the Engineering Department from the Ghent University in 2003. Following up on her studies in computer science, she achieved a PhD in computer science engineering at the Department of Information Technology at the same university on Efficient service management in healthcare. After being a postdoctoral research engineer at the Department of Information Technology, she started as lecturer ICT and ICT research coordinator at the University College West-Flanders. Currently, she is associate professor at Ghent University, IDLab - Data Science Lab.
Her specialties are: multi-sensor and service oriented architectures, novel services, condition monitoring, emotion recognition, machine learning, semantic dashboards, and the fusion of machine learning and semantic technologies, applied in both predictive maintenance and predictive healthcare.
Published on:
13 May 2019