Artificial intelligence, or AI, is all the rage again. Some people – most of them technologists – are looking at AI as a way to resolve some of the problems we face. But others are afraid of it. How can we make sure that AI systems – such as robots – will really help us and not take over the world and snatch our jobs away from us? Pieter Ballon, director of SMIT (an imec research group at VUB), emphasizes that engineers and social scientists need to work together on AI, because artificial intelligence is a technological innovation that will undoubtedly cause significant economic disruption and social changes.
AI is an evolution, not a revolution
Science fiction films featuring robots or intelligent machines in the leading roles (such as Blade Runner, Real Humans, Westworld, etc.) have caused us to look at a future with AI with some trepidation. But it won’t happen overnight and we will also have time to adjust ourselves to the idea and to control AI systems where necessary so that it becomes a gradual evolution, not a sudden revolution. But it is definitely an evolution that is already underway.
Harvard professor, Michael Porter, sets out four stages that mark the way toward smart objects and systems. Stage one is ‘Monitoring’: by using sensors, a smart product will be aware of its own situation and the world around it. An example of this is the Medtronic glucose meter, which uses a subcutaneous sensor to measure a patient’s blood-sugar level, alerting the patient 30 minutes before that level reaches an alarming status.
Stage two is ‘Control’: thanks to its in-built algorithms, the product will then carry out an action based on the readings or measurements it has taken. For example, if a smart camera detects a car with a specific number plate, the gate will open.
Systems then evolve towards the stage of ‘Optimization’. Basing itself on all the data that the system collects while it is operating, in-built algorithms can carry out analyses to determine the best way of working. It’s as though the system ‘learns’ to work more efficiently. An example of this are wind turbines that are able to adjust the position of their vanes each time the wind changes direction so that they can capture a maximum amount of wind energy and also ‘disturb’ the flow of the wind to any neighboring wind turbines as little as possible.
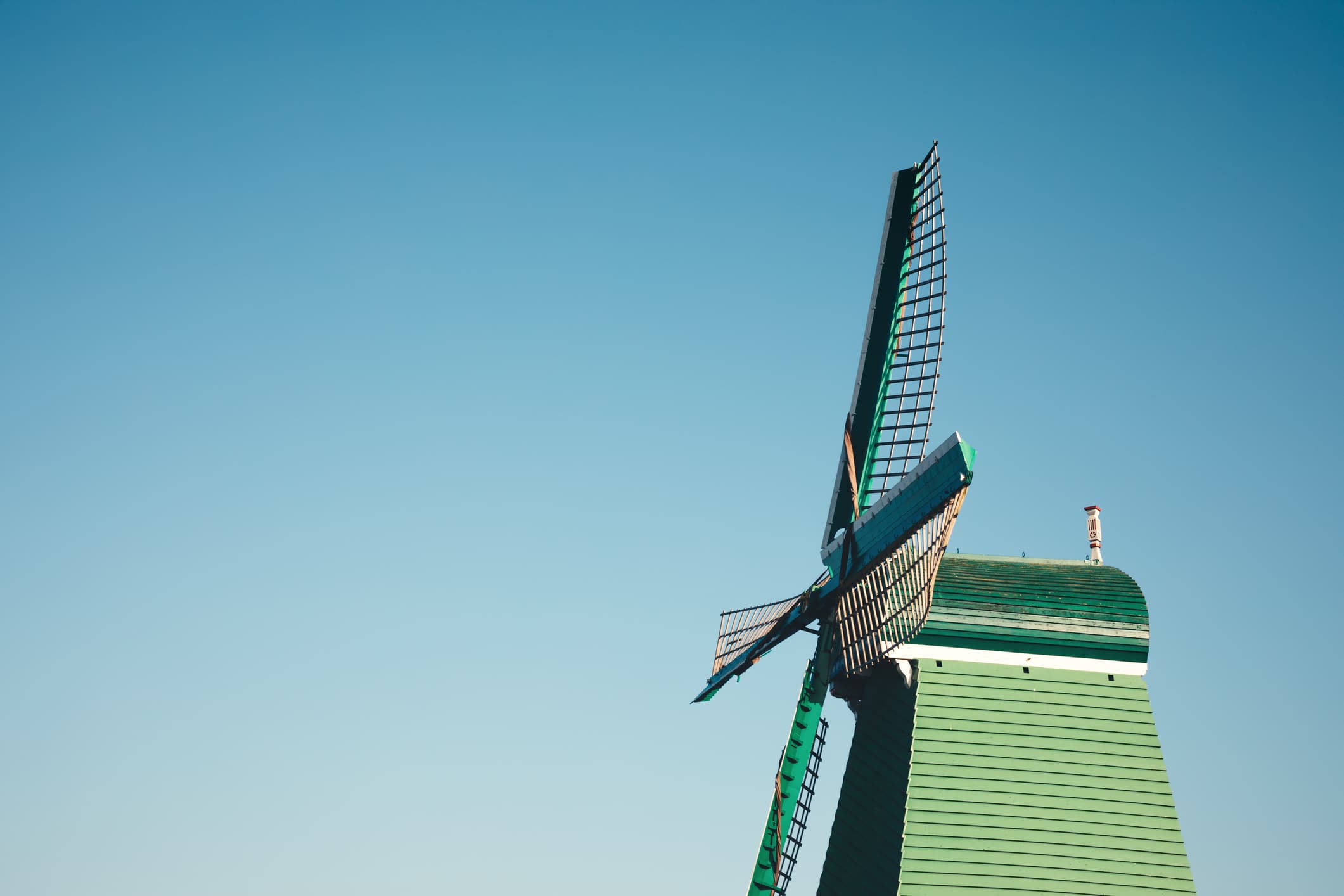
Today's wind turbines not only look very different from their ancestors, they are also much smarter: they can adjust the position of their vanes each time the wind changes direction so that they can capture a maximum amount of wind energy and also ‘disturb’ the flow of the wind to any neighboring wind turbines as little as possible.
Finally, smart systems evolve towards ‘Autonomy’. When a product is capable of monitoring itself or carrying out an action – and making that action as optimal as possible – it can work autonomously. For instance, there is the iRobot vacuum cleaner robot, which is capable of cleaning all sorts of surfaces in the home, as well as detecting dirt, finding its way round furniture and avoiding tumbling down stairs. It also ‘stores’ details of the layout of a room in its memory for the next time and makes its own way back to its recharging station, where it announces its safe arrival with a triumphant sound signal!
Smart systems can also be connected with each other so that they can carry out actions in tandem, learn from each other – and so on. An example of this is the idea of driverless cars and the road infrastructure working together so that if there is an accident somewhere, cars further away from the incident can be notified and the appropriate action taken.
As we can see from these examples, we will gradually evolve towards systems that are capable of learning and taking decisions by themselves. And equally gradually, we – humans – will hand over the monitoring, control and optimization, partly or in full, to machines. This will happen faster in some sectors than in others – and there are various reasons for that. In the mining industry, for instance, Joy Global’s Longwall Mining System is used to dig underground virtually automatically, without any human input. Staff sitting in the control room above the ground keep a close eye on everything going on and only send engineers below ground if it becomes necessary.
So, for the sake of people’s safety, mining has evolved to the most advanced stage of autonomy – although people are still very much a crucial factor of operations.
People and AI systems will become workmates
Human-like AI, human-centric AI, human-in-the-loop AI – these are all terms to indicate that human beings are still very much central to the story. Robots and machines need to be made in such a way that people can understand them, are able to communicate with them and can work efficiently with them. That way, machines can carry out tasks on behalf of and for the benefit of humans.
A good example of this is the ‘cobot’, or collaborative robot, developed to assist Audi production line workers in assembling cars. Whereas previously these types of machines used to be placed in safety cages, the cobot is able to carry out certain actions safely close to and with the help of its human workmates. This means that tasks such as applying adhesive can be carried out much more precisely, consistently and always in the same way. Meanwhile, the cobot’s human workmate is able to control and direct it using hand gestures.
There are still many challenges to overcome in the area of communication between robots and humans. For example, will a robot ever be capable of identifying our intentions?
Can a robot detect if we say something in a fearful or more self-assured way? In which case, this can be important in certain situations. Or when we carry out an action, what does this say about our actual intentions? For instance, it is no easy task to get a driverless car to recognize whether a pedestrian intends to cross the road, or is simply standing at the side of the road. Typically, as a pedestrian, we will try to make eye contact with the driver to indicate that we would like to cross. But this type of ‘unwritten rules’ in human-to-human communication is not easy to transfer to AI systems.
The way humans and cobots work together on the workfloor can take the form of the human demonstrating how something is done and the cobot learning from it so that it can then perform a particular action perfectly. In a single, repetitive process it may be that the human worker will only have to show the robot how to do something a few times and the robot will then take it from there.
But in more complex situations, the cobot may always need a human workmate on hand to give it instructions and to instruct it how to do things. One example of this would be collecting waste in a city. It can be a complex business distinguishing what is waste and what isn’t. It’s also hard to know how to react if someone waves to the waste truck driver and then runs up behind with a bag of waste to be picked up. A robot would not know how to respond, whereas a human knows that the friendliest thing to do is wait.
This means that in some situations, humans and robots will always have to work together, with the robot taking on the heavier work and its human workmate having more time for interaction with other people and knowing how to respond to unexpected situations.
AI systems need to be tested regularly
As we have already said, the human always needs to understand how a robot arrives at a certain conclusion or action – and must always be able to make adjustments where necessary. Recent examples of problems with artificial systems have demonstrated exactly that.
For example, there is the instance of the chatbot Tay, which began posting racist messages on Twitter after certain other Twitter users left politically incorrect posts. The chatbot had not been given any instructions to recognize these types of statements as being inappropriate.
‘Norman’ also made the news in 2018. Norman is an AI system that displayed psychopathic characteristics when doing a well-known test with Rorschach inkblots. It happened because Norman had previously been shown mainly sensational and violent images from Reddit and he had built up a picture of the world based on those images. MIT researchers wanted to use the experiment to demonstrate the danger of ‘false data’ being used as input for AI systems.
And finally, there is also the example of the COMPAS algorithm that was used by the judicial system in America to make predictions about the recidivism of convicts. What happened? Based on the historical data used as input for the algorithm, it reached the conclusion that blacks were more likely to re-offend than whites.
This is Norman, the ‘psychopathic’ AI algorithm that MIT scientists trained to demonstrate the danger of AI when ‘false’ data is used as input. (copyright “Thunderbrush on Fiverr”, https://www.fiverr.com/thunderbrush). At right is one of the Rorschach inkblot tests that Norman was given to look at. In it, he saw a man knocked down and killed by a speeding car, whereas standard AI systems see it as a close-up of a wedding cake on a table.
All this has taught us that we need to look very carefully at the data used as input for AI systems. It also shows us that it is important for us to understand at all times how an algorithm arrives at a certain conclusion – and that we can make adjustments to it. The AI system cannot be a ‘black box’. New procedures and checks are required to ensure data and algorithmic transparency.
Yet it will probably not be possible to make all data input and algorithms transparent – which means there will be times when we do not know why an AI system may have come to certain conclusions. So it is important to introduce regular tests for AI systems, which include paying a great deal of attention to ethical issues, so that any problems can be identified quickly.
How can we trust ‘them’?
So, how can we ensure that everyone is able to trust the AI system they come across on the workfloor, at the doctor’s surgery or on the road? First and foremost by allocating some sort of approval certificate, based on a regular audit of the system. One example of this are the elevators that we use every day. We have no idea about how their technology works, but we trust them to be safe and work properly (and we can do this because they are checked regularly by people who know what they are doing).
The way humans and AI systems communicate and their predictability can also help to build a relationship of trust. For instance, take the traffic lights that we use to cross the road as pedestrians: they have a pushbutton that enables us to provide our own input (while a camera should also be able to detect the pedestrian anyway); we then receive a signal that our input has been registered; next, we wait patiently because we know that we are using a predictable system that will turn green within a maximum of 2 minutes; and in some cases, the system even tells us how long we will have to wait before crossing.
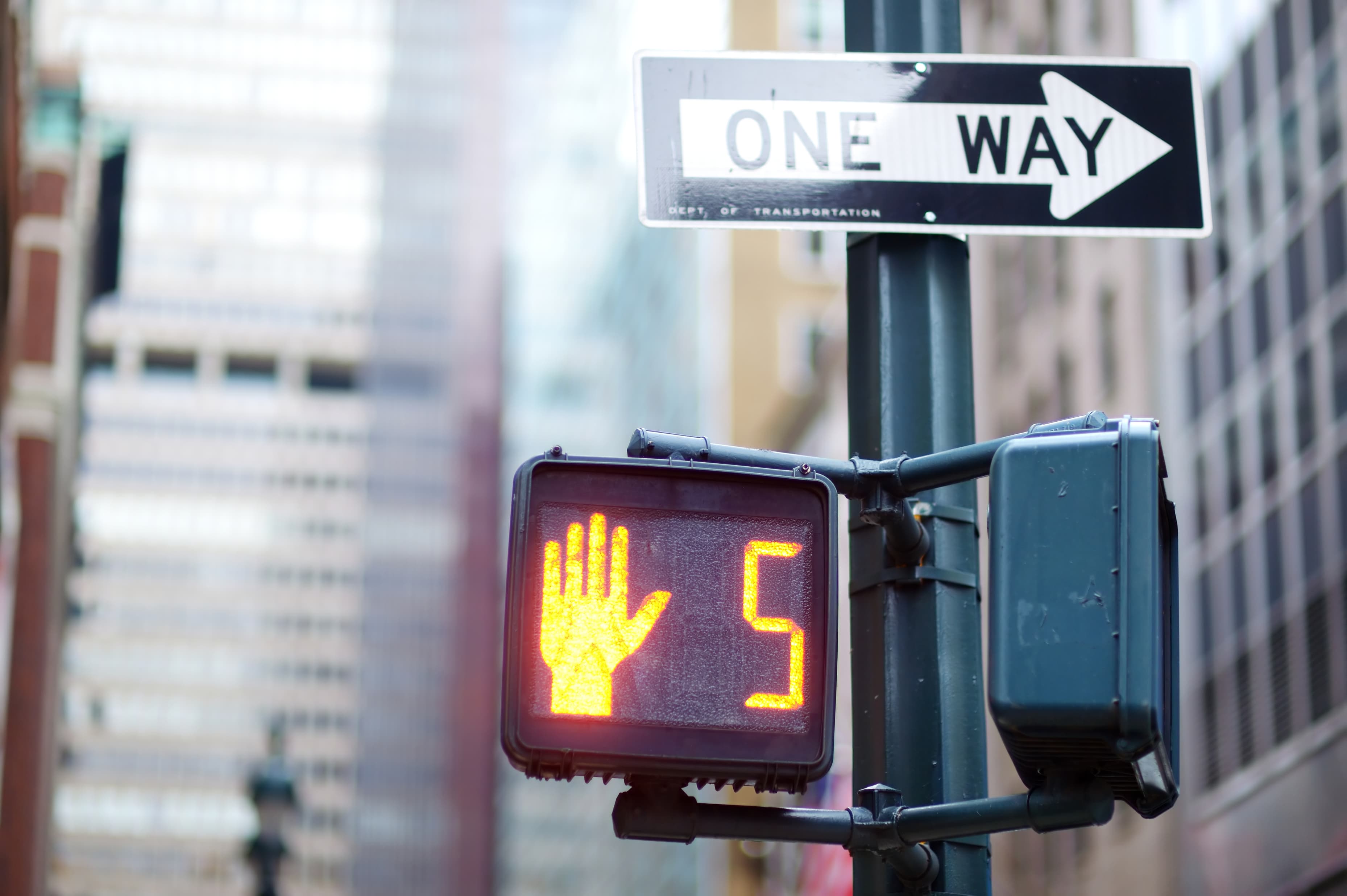
We need to try and achieve the same level of trust in AI systems that we already have in smart traffic lights.
Will we still have a job in 2035?
There is a good chance that many routine jobs will be taken over by AI systems and robots. That may range from working on a conveyor belt in a factory, to making certain medical diagnoses or working as an accountant or in legal jobs.
Even tasks where a bit more creativity is required can be carried out by AI systems. The well-known historian, Yuval Harari, quotes the example of Google’s AlphaZero, which took less than 4 hours to learn how to play chess, after which it was able to beat the best human-trained chess computer. Not by learning from historical data, but by using machine learning to teach itself to play the game. This has gone so far now that when players in chess tournaments make a move that is strikingly creative and original, the judges may suspect that player is using a chess computer to come up with the moves.
So, both routine jobs and jobs in which new possibilities have to be explored can be carried out by AI systems. But will they ever be as creative as we, humans? That remains to be seen.
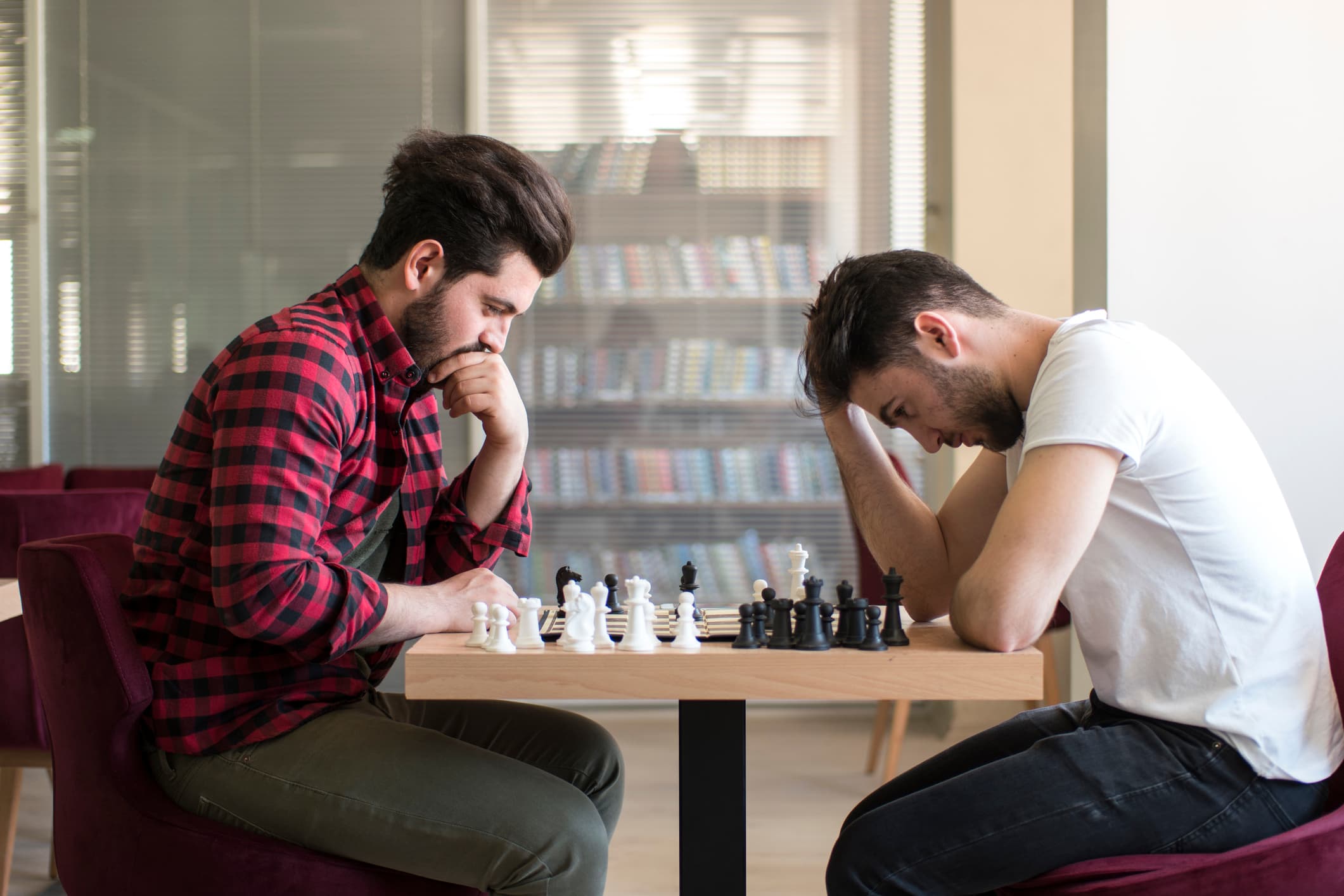
Chess computers are a great example of what is possible with AI. They have gone so far that players can be suspected of using chess computers if the moves they make are strikingly original and creative.
Whatever happens, the content of many jobs will be changed by the arrival of AI. We will work with these AI systems and have to keep adjusting to new capabilities. Lifelong learning will be very important, both for low-skilled and highly qualified jobs. New types of job will also be created that we can’t predict at the moment. Typically, jobs where human contact is very important – such as nursing – will still be done by humans, even though robots may be brought in to provide assistance and support.
But the impact goes further than just our job content and we will also find other economic models becoming involved in which we will work less and in which job, income and consumption will be separated out from one another.
So perhaps you won’t have to work (fulltime) to receive a full income – and maybe you won’t need a full income to provide for your basic needs.
Just look at the current digital economy of apps and digital services. You can use many services free of charge and sometimes (with or without you realizing it) in exchange for access to your data. With this system, data will become a new tradeable commodity and so we will be able to ensure better that our data is our own property and that we can use it transparently when and where we want.
How is imec contributing to this future?
Imec is working on neuromorphic chips that are able to support complex algorithms efficiently and without consuming too much energy. Being economical is important when it comes to building AI into sensors. Imec is also involved in the ExaScience Life Lab to build supercomputers for major medical problems (developing new medication, understanding diseases better, etc.).
The imec research group SMIT set up the internal DANDA project to make AI more transparent en to make the algorithms understandable for different parties involved. Methods such as post-it data flow mapping and a description of the data preparation process were developed for imec’s AI developers. Also, the DELICIOS project was launched in 2018. This four-year project will see researchers examining which complex tasks humans will be willing to transfer to autonomous systems and on what terms – as well as how comfortable they feel about it. Trusting these systems will be one of the central points in the study.
A technology such as AI cannot be developed without taking account of the social and economic implications from the outset. For this reason, imec will be intensifying this multidisciplinary research in 2019.
This article is part of a special edition of imec magazine. To celebrate imec's 35th anniversary, we try to envisage how technology will have transformed our society in 2035.
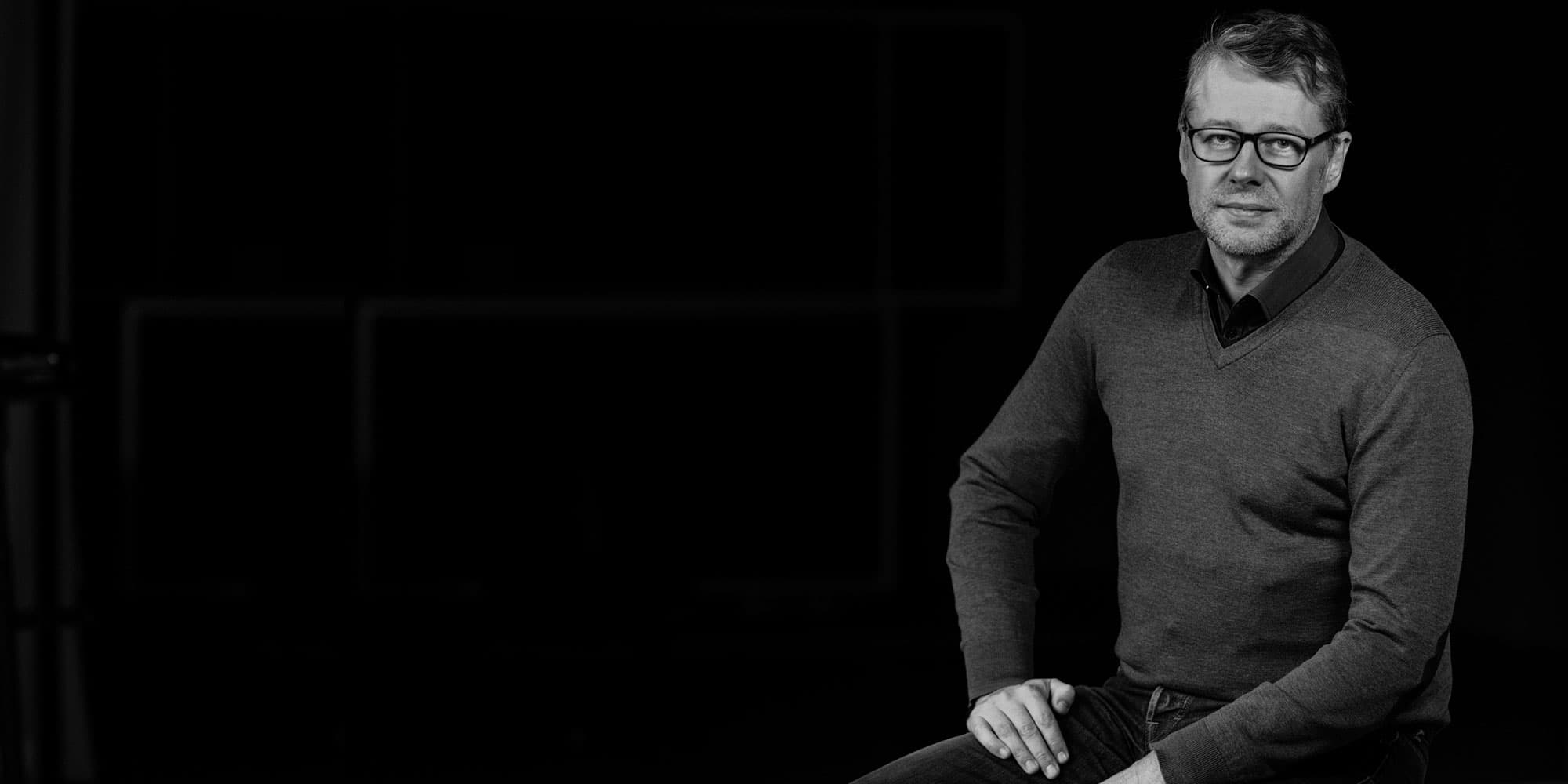
Professor Pieter Ballon gained his master’s degree in Modern History from KU Leuven and his PhD in Communication Sciences at VUB. He has taught Communication Sciences at VUB since 2009. Since 2016, he has been director of SMIT, an imec research group at VUB focusing on ‘Studies in Media, Innovation and Technology’. Pieter Ballon was appointed the first Brussels Smart City Ambassador and is also the International Secretary for the European Network of Living Labs. His publications include the book “Smart Cities: how technology keeps our cities livable and makes them smarter”.
Published on:
4 January 2019