In the last decade, differentiation has been the buzzword in education, yet also its biggest challenge: how can a single teacher address the needs of over 20 pupils – from varying backgrounds and with diverse cognitive levels and interests – all at the same time? Luckily, artificial intelligence will come to teachers’ aid. By 2035, children will be able to follow a completely personalized learning path, adapted not only to their skills, but also to their preferences and interests. In this article, dr. Jung Yeon Park, researcher in psychometrics and educational statistics at ITEC, an imec research group at KU Leuven, shares her view on how technology will transform the classroom.
There is no such thing as ‘one-size-fits-all’
Do you remember that training course where you stopped paying attention after about half an hour? Chances are that you zoned out because what the teacher was saying was either too difficult, too easy or irrelevant for you. Intuitively, we all know that learning works best if it addresses our individual needs. But in the daily reality of our children’s classrooms – with one teacher for about 20 pupils – personalization is hard to accomplish.
Most of the time, everyone sits in the same classroom and receives the same input, targeted at an imaginary average student. Not only does this affect their learning efficiency, it also has a negative impact on children’s attitude towards school. Weaker students get frustrated or suffer from low self-esteem whereas stronger students get bored waiting for more challenging tasks. Teachers try their best to cater to individual needs, but this is not an easy task. So how can we ensure that students get personalized education without expecting teachers to magically evolve into superhero jugglers?
Sneak peek into the classroom of 2035
Let’s fast-forward to a hypothetical classroom in 2035. When class starts, each child goes to work on his/her own device and logs onto his/her personalized learning platform. Tom’s dashboard suggests that he does some exercises on mathematics or practices the conjugation of French verbs. He decides to start with French because in the afternoon they will be working on their creative project in French. The platform suggests that he watches a short video lecture first, but he decides to skip it. He prefers a more trial-and-error-based approach and – because the learning content is presented in the format of a game – he is eager to start practicing.
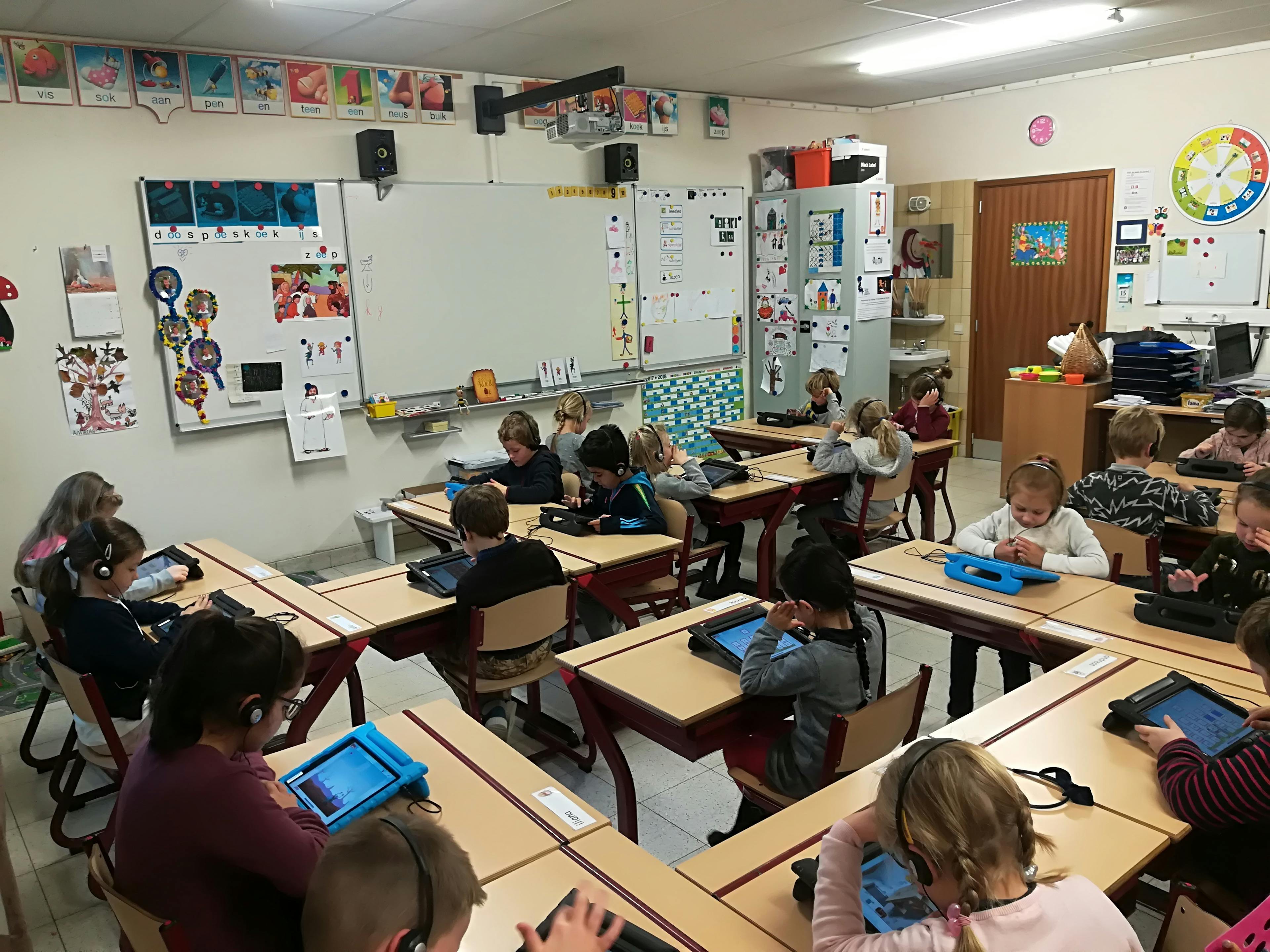
Children playing an adaptive learning game developed as part of the imec.icon LEAPS R&D project. Although they all play the same game, each child is presented with learning content adapted to his/her personal needs.
French is not Tom’s strong suit. Last time he tried to play the French conjugation game, he did not do very well. To improve his proficiency, he has been watching a French crime series in the last few weeks. At the start, the platform presents him with exercises that are actually a bit too easy for him, but the system quickly picks up that his level has improved and starts to present more challenging content. Tom notices that some of the phrases seem to have been taken from the series he watched. The teacher walks around the classroom to help students who need extra explanation. She can easily see who is struggling thanks to her augmented reality (AR) glasses: when she fixes her gaze on a particular student, she gets to see an overview of their progress.
Sometimes Tom is allowed to log onto the school platform from home, but today they have to work on their creative group projects after the break. In a society in which artificial intelligence has become mainstream on the work floor, developing soft skills – such as creativity, communication, team work, empathy, etc. – is very important. To work on the project, Tom and his partner switch on their AR glasses. The AI in the glasses spontaneously selects and projects visuals to support their conversation, e.g. a map of the region they are discussing. Meanwhile, the teacher walks around the classroom and checks their progress, also evaluating how they are working together and steering them in the right direction if they get stuck.
Artificial intelligence that ‘knows’ you
In the last 10 years, learning analytics – the science of measuring, collecting, and analyzing data on learning progress - has become an increasingly important field in educational research. If you have enough big data on a student’s learning process, artificial intelligence can use this to create a personalized – i.e. adaptive – learning experience.
How does adaptive learning work today? A self-learning system that analyzes how you respond to the tasks presented and – depending on your performance – selects new tasks that address your respective strengths and needs. It also continuously re-evaluates your level, so – just like in Tom’s case – it will notice if you have made progress in-between online learning sessions, e.g. by engaging in other learning activities like studying, remedial lessons, etc.
By 2035, adaptive learning systems will become much more advanced, taking into account not only educational needs but also personal preferences. Some people prefer to study the theory first before doing exercises, whereas others prefer to dive right in and figure out the rules as they go. Today the teacher’s personal preference often determines which learning pattern is dominant in the classroom, but by 2035 adaptive learning systems will be able to recognize which learning method feels most natural to you. A second improvement that we can expect in the near future is the ability to track proficiency across domains and subjects. Data on your mathematical skills could be useful to learn about chemistry, for instance. The system will also be able to generate and evaluate complex tasks, like writing assignments and providing feedback tailored to students’ individual personalities and needs.
As the technology matures, adaptive learning will become an essential tool to reduce teachers’ workload and enable differentiation. Adaptive learning platforms will become a widely used tool to support education – the way textbooks are today – but artificial intelligence can also come in other shapes and sizes, from wearable technology (AR glasses, cyber suits, smart watches, etc.) to even robots.
Several schools are actually already experimenting with ‘social’ robots that can interact almost like humans. They can even ‘read’ faces to recognize emotions and mood. Research has shown that these kinds of robots can be even more efficient than software, because of their social presence (almost like learning with a friend). Robots could function as teacher assistants who work with students one-on-one. The robots do not necessarily have to be all-knowing geniuses, because that could make them too intimidating. To make them more ‘human’, they can be programmed to occasionally make mistakes. In fact, one promising research track is to make the robots ‘dumb’ and let the children learn by teaching the robot how to write, how to do multiplications, how to speak French, etc.
When emotions come into play
Motivation – but also stress levels, cognitive load and learning engagement - play a crucial role in the learning process. Understanding how someone feels when learning could be very valuable information to optimize adaptive learning systems. For instance, if the system detects that the learner’s attention is dwindling, it could present a different kind of learning activity better suited to the learner’s preferred learning strategy. Or if it notices that a student is particularly demotivated on a given day, the system could decide to present a less challenging learning path adapted to the learner’s mood.
In a way, it is a bit similar to what teachers intuitively do when they get the impression that students are struggling to pay attention. Only – unlike teachers who have to cater to the whole group at the same time – an adaptive learning platform can provide a unique approach for each individual student.
The difficulty is of course that these constructs – i.e. motivation, stress, cognitive load and learning engagement – are very hard to measure. Researchers are currently exploring several tracks to match physiological signals (e.g. brain waves, electrodermal activity, cardiovascular) from wearable physiological sensors with self-reported information. By 2035, we will probably be able to unobtrusively monitor students’ stress levels, cognitive load, engagement and motivation in real-time thanks to advanced wearable technology, but we should of course be careful with this kind of information. Do we really want teachers to know in-real time how their students feel? And how should they use it? Do they always have to react when students are distracted or feel very stressed? Of course, the last thing we want is to make students stressed simply because we are measuring their stress levels.
How is imec contributing to this future?
Driving innovation in education is about more than just technology. Although EdTech has been around for a while, its implementation in mainstream education has been slow. For too long, educational technology has been developed in a cocoon outside the educational context. That’s why collaboration with teachers and other stakeholders is a key principle in imec’s Smart Education program.
Ideally, innovation comes from teachers themselves. That is why imec launched Smart Education @Schools, an instrument for open innovation set up in collaboration with the Flemish Government. The idea is that schools work together with universities, research centers, companies, and other partners from the field to explore their own innovative tech-related ideas. The projects submitted by schools this year were very diverse, ranging from smart wristbands for kindergarten pupils, to VR environments for traffic education, and chatbots to improve study skills.
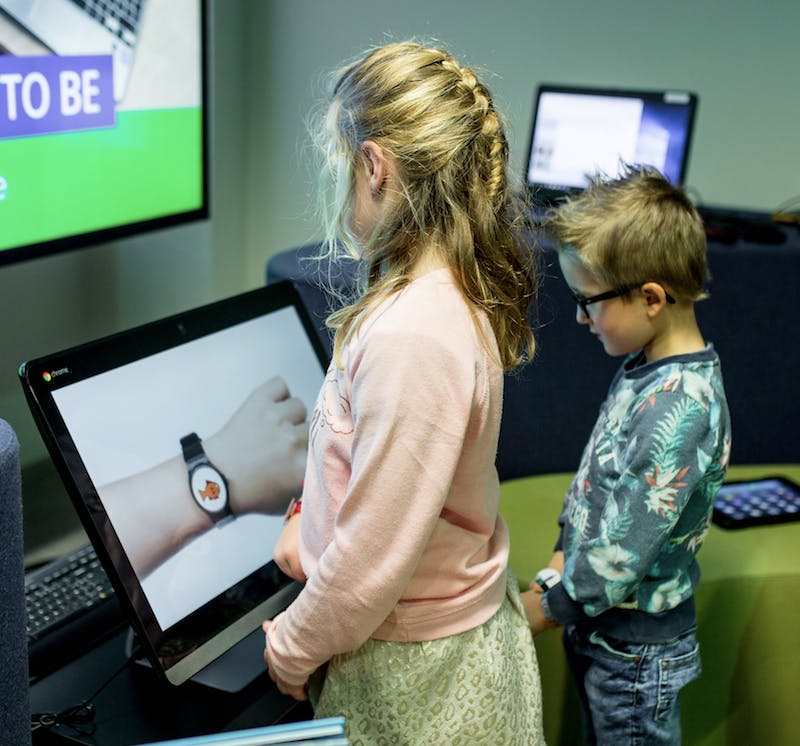
One of the Smart Education@School projects explores how smart wristbands can be used to help teachers get better insight into each child’s talents and interests.
In 2018, in collaboration with industrial partners and schools, imec developed a new self-learning adaptive learning system (LEAPS) that is generic enough to be used in any context. Whereas many existing adaptive learning platforms still require a domain expert to decide on the difficulty level of an exercise, the self-learning LEAPS system can do this entirely autonomously and on-the-spot. This makes creating new adaptive learning applications a lot less cumbersome and expensive, making it easier to implement them in a wider educational context.
Another issue that is currently a hot topic in adaptive educational games is the complex relation between gaming skills and cognitive skills (e.g. calculus). Suppose a child is playing a calculus game where he/she has to shoot a rocket at the correct answer. What if the child is good at calculus, but bad at shooting rockets? Currently, this would imply that the learner only gets easy exercises, which means that – for this learner – the game would lose its educational value. At imec, we have recently developed a new technique for multidimensional skill monitoring, enabling the system to distinguish between the relevant cognitive skills (related to the learning objective) versus their gaming skills.
Want to know more?
- Find more information on imec’s Smart Education program or discover what’s new at ITEC, an imec research group at KU Leuven specialized in smart education.
- To find out more about the self-learning adaptive learning system developed as part of the LEAPS project, you can download the leaflet here or watch this video.
- For more information on imec’s Smart Education @ Schools project, check this website (in Dutch).
- Researchers from IDLab, an imec research group at UGent, are exploring the potential of social robots in education. Interested to know more? Check this article in Science Robotics.
- Imec is collaborating with medical experts to uncover the link between physiological signals and stress, as is explained in this imec magazine article. These insights could also be valuable to monitor student’s motivation and learning engagement.
This article is part of a special edition of imec magazine. To celebrate imec's 35th anniversary, we try to envisage how technology will have transformed our society in 2035.
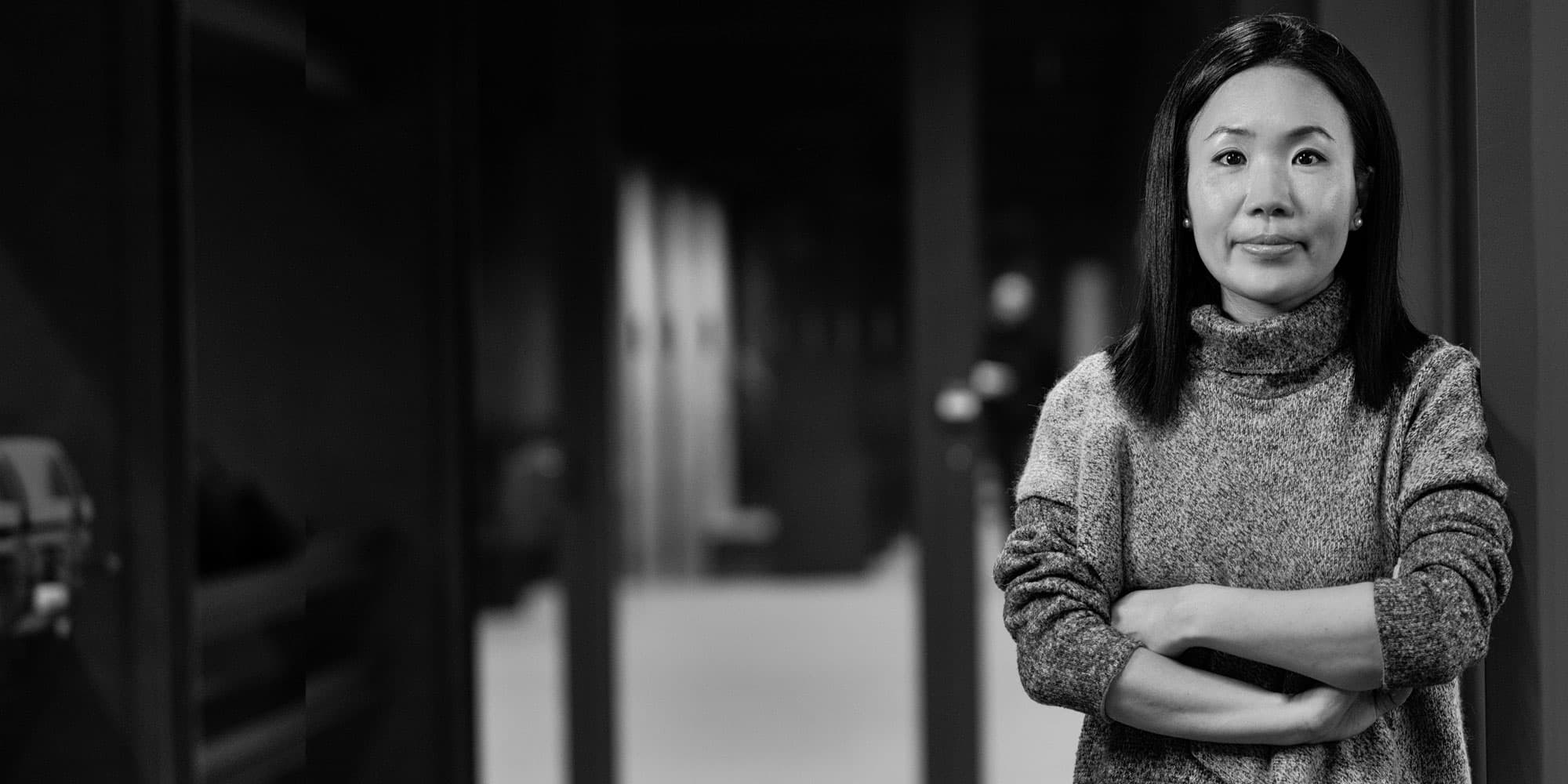
Dr. Jung Yeon Park is a senior researcher in in psychometrics and educational statistics at ITEC, an imec research group at KU Leuven. Her research focuses on the development of statistical methods and data mining techniques to understand causes, patterns, and consequences of educational outcomes in e-learning environments. In 2015 Dr. Park received her doctorate from Columbia University where she was a recipient of the National Science Foundation doctoral fellowship. She was involved in the imec.icon project ‘LEAPS: LEarning analytics for AdaPtive Support’ and also is a member of the postdoctoral management team of imec’s Smart Education Program.
More about these topics:
Published on:
8 January 2019